Журнал высшей нервной деятельности им. И.П. Павлова, 2020, T. 70, № 6, стр. 723-737
Resting-state networks in adolescents with poor behavior regulation. An analysis of effective cortical connectivity in eeg source space
A. V. Kurgansky 1, *, D. I. Lomakin 1, R. I. Machinskaya 1
1 Institute of developmental physiology, Russian academy of education
Moscow, Russia
* E-mail: akurg@yandex.ru
Поступила в редакцию 12.09.2019
После доработки 10.12.2019
Принята к публикации 16.12.2019
Аннотация
The general direction of this study is the search for possible neurophysiological causes of deviant behavior. The specific goal of the work was to assess to what extent the effective connectivity that was estimated in the EEG source space at rest is sensitive to the non-optimal state of several most relevant resting-state networks (RSN) that are closely associated with emotional and motivational control and executive functions in adolescents. Individual records of the background EEG of two groups of adolescents 13–14 years of both sexes (24 girls and 28 boys, 52 in total): control group (30 adolescents) and group of adolescents showing signs of deviant behavior (22 adolescents) were used to assess the strength of effective (directed) connections between the nodes of three resting-state networks: the default mode network (DMN), the salience network (SN) and the central executive network (CEN). It was found that the pattern of effective connectivity between cortical regions belonging to the three CEN, SN and DMN neural networks differed in adolescents from the control group and those in the group with signs of deviant behavior. It was also found that the differences between these two groups were not the same for two sexes and were frequency-specific in nature.
Adolescence is known as the most turbulent period of human developmental course. Adolescents show deviant behavior (violation of social norms) far more often than do younger children or adults and they are more prone to commit impulsive and risky actions and more susceptible to various kinds of addictive behavior, such as gambling [Che et al., 2017] and drug addiction [Caouette, Feldstein Ewing, 2017]. A threefold increase in mortality among adolescents [Casey, 2015] indicates that this pattern of risky and self-destructive behavior is more than merely a hallmark of adolescence but a serious problem that deserves consideration.
In psychological literature, the adolescence-specific behavior is frequently viewed as being related to a reduced ability to self-control (for review and discussion, see [Casey, 2015; Steinberg et al., 2015; Shulman et al., 2016]). This view however is in an apparent contradiction with rapidly developing working memory, inhibitory control, and flexibility – the very abilities usually considered as core executive functions [Diamond, 2013]. It has been suggested that the source of this contradiction may be that the immaturity of self-control mechanisms in adolescents refers only to emotionally colored situations, while the behavior in emotionally neutral circumstances is quite successfully controlled, although the function of cognitive control continues to improve [Casey, 2015]. This explanation is supported experimentally [Botdorf et al., 2017]. In this work, adolescents were evaluated for risk inclination in the Stoplight game (driving a car with stops at many intersections), and the degree of self-control using two versions of the Stroop task: in the emotionally neutral variant (Cognitive Stroop task) and the emotionally colored variant (Emotional Stroop task). The main result of this experiment is that the statistical relationship between the propensity to take risks and the assessment of self-control was found only for the case of the emotionally-colored variant of the Stroop task.
Three neurobiological models accounting for adolescence specifics are considered in the literature (for review, see [Casey, 2015]). The dual-system model of S. McClure and colleagues [McClure et al., 2004] suggests the dominance of the limbic system of emotional (“hot”) control, which provides an immediate positive result or reinforcement (immediate rewards), over the prefrontal system of rational (“cold”) control, which provides a delayed receipt of the desired result (delay rewards). Yet another model is the triadic model of motivated behavior proposed by M. Ernst and colleagues [Ernst et al., 2006]. This model divides the emotional control system into the reward-driven and the harm-avoidance (amygdala-related) subsystems and assumes that adolescence being a time when there is a bias toward the reward-driven system. A third model is referred to as the imbalance model [Casey et al., 2008]. According to this model, regional neurochemical, structural and functional brain age-related changes cause imbalances between limbic and prefrontal control regions which in turn results in specific changes in behavior during adolescence.
Note that common to all these models is a concept of a balance in activity of two or more subsystems competing for overall control over adolescent’s behavior. This is in full accordance with the key theoretical view of the modern cognitive neuroscience which states that the actual functional units underlying psychological functions and observed behavior are not isolated brain modules but ensembles of interconnected brain structures making up the large-scale brain networks [Bressler, Menon, 2010; Menon, 2015; Pessoa, 2018]. Hence the interest in assessing potential and actual interactions among brain structures by evaluating anatomical pathways (structural connectivity), measuring statistical correlation (functional connectivity) and causal influence (effective connectivity) between neuronal activities of those structures. The valuable information about the structure of a large-scale brain network comes from studying functional and effective connectivity within and between resting-state networks [Fox, Raichle, 2007].
It has been shown that, in adolescents, the functional connectivity within and between brain structures closely related to emotional-motivational and cognitive control brain systems is sensitive to such clinical condition as depression [Pannekoek et al., 2014; Bradley et al., 2016; Davey et al., 2017] and shows significant alterations in adolescents prone to excessive risk-taking, gambling, and substance abuse [Qu et al., 2015; Wilcox et al., 2016; Shulman et al., 2016; McCormick et al., 2018].
The goal of the present work was to explore whether effective connectivity between the representative cortical regions belonging to these brain systems related to emotional-motivational and cognitive control in the adolescents showing signs of deviant behavior was different from that of the age-match control group. To this end we estimated the strengths of effective connections computed in the EEG source space in the rest condition for the following three relevant resting-state networks (RSNs): the default mode network (DMN), the salience network (SN), and the central executive network (CEN). The brain structures belonging to these RSNs (prefrontal, cingulate and insular cortex, amygdala) are known to be involved in voluntary control of behavior and emotional-motivational regulation [Catani et al., 2013; Bernhardt, Singer, 2012].
The choice of these three RSNs is due to the fact that the emotional and motivational processes and controlling functions of the brain are closely related to the interaction of brain structures entering these networks [Chen et al., 2013; Esménio et al., 2019]. Note, that it is this interaction that is supposed to be unbalanced in adolescents [Casey, 2015].
The study of such interactions at rest is a widely used alternative to the study of them in the process of activity. Indeed, the structure of the RSN reflects the predominant morphological connections between the brain formations included in it – the network nodes [van den Heuvel et al., 2009; Mišić et al., 2016]. Moreover, the structure of functional and effective connectivity between these formations, estimated on the basis of analysis of electrophysiological signals (EEG/MEG), is in good agreement with that obtained on the basis of analysis of spontaneous fluctuations of the BOLD signal [Sockeel et al., 2016; Yuan et al., 2016].
It follows from the above that if two groups of subjects differ in the strength of the effective connections between the nodes of three RSNs measured on the basis of EEG or MEG, this can be considered as an indication of differences in the structure (including the degree of development) of morphological connections between these nodes. In turn, differences in the structure of morphological connections can affect the effectiveness of the joint work of various parts of the brain in the process of cognitive activity. This approach is experimentally confirmed: it has been shown that patient groups are characterized by altered interactions between RSN nodes at rest [Ho et al, 2015; Franzmeier et al., 2017].
METHODS
Participants. Two groups of adolescents of 13–14 years old of both sexes (24 girls and 28 boys, 52 people in total), all students of the Moscow/Moscow suburb high schools, participated in this study. The group of children with signs of deviant behavior included 22 adolescents (6 girls and 16 boys) who, according to the school psychologist, had behavioral and/or emotional problems at school and when interacting with their parents. A total of 30 adolescents (18 girls and 12 boys) were in the control group.
All adolescents and their parents gave informed written consent to participate in the study.
EEG collection. We recorded high-density EEG in subjects who were at rest with their eyes closed (hereinafter referred to as background EEG). EEG was recorded at a sampling rate of 250 Hz using a NetStation software (Electrical Geodesics, Inc.) in the frequency range 0.1–70 Hz from 129 electrodes located on the HydroCel GSN, with vertex as a reference.
EEG preprocessing. Individual records of the background EEG were extracted from the files created by the NetStation and converted to the mat-format for subsequent processing in the Matlab/Octave environment. These individual recordings were visually inspected for the presence of bad channels and artifacts which were manually marked and then removed during further signal processing.
EEG source estimation. The estimation of cortical sources of background EEG was performed in the approximation of distributed sources [Darvas et al., 2004], with the dipole orientations being fixed to be orthogonal to the surface of the cortex [Litvak et al., 2011]. In this approximation, the electric potential at each electrode at an arbitrary time is determined by the weighted sum of the partial contributions of current dipoles located in small volume elements (voxels) of the cortical tissue and having fixed direction in space. All calculations were carried out in the Matlab/Octave software environment using functions of SPM8 software [Litvak et al., 2011] and a custom implementation of eLORETA algorithm [Pascual-Marqui et al., 2011]. In the above computations, we relied on the averaged coordinates of the HydroCel GSN sensors (www.egi.com) and the standard geometry of the skull and brain (ICBM-152).
Regions of interest. The effective connectivity was assessed for several preselected cortical areas – regions of interest (ROI). Following [Chen et al., 2013], we chose the following 6 areas belonging to three RSNs (the MNI coordinates of the centers of these areas are given in square brackets). For CEN, the right lateral prefrontal cortex (dLPFC: [38, 16, 50]) and a portion of the right lateral parietal cortex (LPC: [45, –64, 40]) were selected. For SN, the dorsal anterior cingulate cortex (dACC: [1, 9, 52]) and the right fronto-insular complex (FIC: [49, 13, –6]) were selected. Finally, for DMN, the medial prefrontal cortex (MPFC: [–2, 53, 5]) and the posterior cingulate cortex (PCC: [–1, –61, 16]) were chosen. Each region of interest was assigned a single signal (one time series), which characterizes activity of all voxels enclosed within a 1 cm radius of the sphere, built around the center of this region. We subjected activities of these voxels to the Principal Component Analysis (PCA) and used the first principal component (the component with the highest variance) as a signal specific to the particular ROI. The percentage of the variance explained by the first principle component varied from ROI to ROI, from segment to segment and from participant to participant, with 95% of all values of this value lying in the range from 45% to 99% (its average value was 83.8%). Thus, in the source space, the activity of selected ROIs was characterized by 6 time series.
Estimation of effective connectivity. To assess the strength of cortical-cortical effective connections we used a vector autoregression (VAR) which is a linear method modeling directed influence of multiple channels [Kurgansky, 2010]. We fitted a 6-channel model of 14-th order to EEG data.
The VAR model accounts for the statistical structure of a multichannel signal based on the assumption that at an arbitrary discrete point in time n the $x\left( n \right)$ = {x1, …, xM}T column vector (T superscript corresponds to the transposition operation) formed by the signal samples taken from М channels is determined by a linear combination of the past samples of this signal up to some residual white Gaussian noise:
(1)
$x\left( n \right) = \mathop \sum \limits_{p = 1}^{p = P} \,a\left( p \right)x\left( {n - p} \right) + e\left( n \right).$In expression (1), the upper summation limit P corresponds to the model order, i.e. the least recent samples participating in the above expression; the sequence $a\left( p \right)$ of square МxМ matrices contains the unknown autoregressive coefficients ${{a}_{{{\text{km}}}}}\left( p \right)$ to be determined.
In the frequency domain, the M-channel VAR model is reduced to a simple multiplication of matrices
Here, capital letters $X\left( f \right)$ and $E\left( f \right)$ denoted Fourier transform of $x\left( n \right)$ and $e\left( n \right)$ vectors, respectively. The elements of $H\left( f \right)$ matrix are linear frequency filters that determined by the coefficients of $a\left( p \right)$ matrices. Thus, the VAR model states that the signal observed in each channel is generated by mixing the outputs of М frequency filters, each fed with independent white Gaussian noise as its input.
The spectral matrix containing auto- and cross-spectra is expressed in the following way through the matrix and the covariance matrix of noise:
(H superscript means Hermitian conjugate). It follows from (3) that if white Gaussian noises in different channels are statistically independent then the signal in each channel is the sum of the independent contributions of all M channels:(4)
${{S}_{{{\text{kk}}}}}\left( f \right) = \mathop \sum \limits_{m = 1}^{m = M} \,\sigma _{{{\text{mm}}}}^{2}{{\left| {{{H}_{{{\text{km}}}}}\left( f \right)} \right|}^{2}}.$The degree of influence of one channel on another (directed influence) can be conveniently estimated using the directed coherence function (directed coherence, DC, first suggested in [Baccala et al., 1998]) computed according to the formula
(5)
${\text{D}}{{{\text{C}}}_{{{\text{mk}}}}}\left( f \right) = \frac{{{{\sigma }_{{{\text{kk}}}}}{{H}_{{{\text{mk}}}}}\left( f \right)}}{{\sqrt {{{S}_{{{\text{mm}}}}}\left( f \right)} }}.$As follows from the above formula, at a given frequency f and for any pair of channels with indices k and m, the DCkm( f ) function shows which fraction of the signal power observed in the k-th channel is explained by the contribution of the m-th channel.
It should be pointed out that the overall duration of artifact-free EEG generally varies from subject to subject and so does the duration of the corresponding signals in the source space. If the DCkm( f ) function is computed using autoregressive coefficients found for signal records of different lengths, the function will be subject to statistical bias – the shorter the record, the greater the bias [Kurgansky, 2010]. In order to circumvent such a bias, for all subjects, we estimated the VAR model coefficients from the signal segments of the same length, which ensured the same statistical bias value for all individual data. This was achieved as follows. For each subject, the source space activity corresponding to the artifact-free EEG was divided into non-overlapping segments of one second duration. Then, the entire set of the segments was used to arrange sequences of 10 segments each. The number of these sequences which equals a total number of segments divided by modulus of 10 was of course different for different subjects. A separate VAR model was fitted to each 10-segment sequence. Since individual segments in such a sequence did not constitute a continuous recording we used a special algorithm that fit a single VAR model to a set of multichannel EEG segments of equal length [Cui et al., 2008]. For each of the VAR models fitted to 10-segment sequence, the corresponding DCkm( f ) function was computed. Each subject was characterized by the DCkm( f ) function averaged over all partial VAR models.
Statistical analysis. The values of this function were further analyzed in six frequency ranges: theta (4–7 Hz), alpha-1 (9.5–10 Hz), alpha-2 (10–13 Hz), beta-1 (14–18 Hz), beta-2 (20–27 Hz) and gamma (30–40 Hz). For each pair of channels (pair of ROIs), we computed six values by averaging the corresponding DCkm( f ) function over each of the six frequency ranges. The statistical hypotheses were tested using repeated measures analysis of variance (rmANOVA).
This analysis design was applied separately to three subsets of effective connections. We analyzed (1) all links connecting CEN (dLPFC, LPC) and SN (FIC, dACC), (2) all links connecting CEN (dLPFC, LPC) and DMN (MPFC, PCC), and (3) all links between SN (FIC, dACC) and DMN (MPFC, PCC) resting-state networks. Note that we excluded from the analysis all effective connections between nodes within the same network as well as “self-influence” – the directed influence of nodes on themselves.
In each of those three analyses, within-subject factors included the frequency range FR (6 levels), nodes of the first resting network LOC1 (2 levels), nodes of the second resting network LOC2 (2 levels) and the direction of effective DIR interaction (2 levels: “direct” from LOC1 to LOC2 and the “inverse” from LOC2 to LOC1). Naturally, this is a matter of arbitrary convention, which network is considered the first and which is the second. Below the “first” is always considered the network that is mentioned first in the verbal description. So, in the first subsection “Connections between CEN and SN” of the Results (see below), the CEN network is considered the first. Similarly, when describing the results, the phrase “connection between the node b of the network B and the node d of the network D” should be understood as the directed influence of b on d, which we will also denote b → d.
Between-subject factors in all three cases included the GR group (2 levels – control and deviant) and the GEN gender (2 levels).
We illustrate the meaning of the factors LOC1 and LOC2 by the example of directional connections between nodes belonging to the central executive network (CEN) and the salience network (SN). Each of these networks includes one pair of areas of interest (nodes): for CEN, these were dLPFC and LPC, and for SN, these were FIC and dACC. Then the factor LOC1 has 2 levels, which correspond to signals in one of two CEN nodes: dLPFC (LOC11) or LPC (LOC12). The LOC2 factor also has two levels that correspond to signals in one of the FIC nodes (LOC21) or dACC (LOC22). Thus, the four “direct” (LOC1 → LOC2) effective connections between these networks are as follows dLPFC → FIC (LOC11 → LOC21); LPC → FIC (LOC12 → LOC21); dLPFC → dACC (LOC11 → LOC22); LPC → dACC (LOC12 → → LOC22).
It would seem that instead of a pair of LOC1 and LOC2 it is enough to use one “PAIR” factor with four levels corresponding to the four listed relationships, however, using a hierarchical scheme with factors LOC1 and LOC2 has its own advantage. It makes it possible to take into account not only the directed influence of a node of one network on a node of another, but also the directed influence of one network as a whole on another as a whole (the combined effect of a pair of nodes of one network on a pair of nodes of another network), as well as the general effect of one network on individual nodes other.
RESULTS
In this section we report the results obtained sequentially for all three schemes of statistical analysis. In addition to the description of these results in the text, they are also illustrated in Figure 1, which schematically shows all the effective relationships that are significantly different in adolescent prone to deviant behavior (DGRP) and in adolescents in the control group (CGRP).
Fig. 1.
Effective connections showing significant differences between adolescent with signs of deviant behavior (DGRP) and control group adolescent (CGRP). For each of the six frequency ranges (θ, α1, α2, β1, β2, γ), three RSNs (CEN, SN, DMN) are shown as ovals. In each oval there are two rectangles representing two nodes (two cortical regions) of the corresponding RSN. Arrows indicate directed interactions (effective connections) between RSNs. Arrows can begin on the contour of the oval (the whole RSN affects its counterpart) or on the rectangle inside the oval (a single RSN node affects its counterpart). Arrows can end on the contour of the oval (the whole RSN is affected by its counterpart) or on the rectangle inside the oval (a single RSN node is affected by its counterpart). Solid arrows indicate the directional links whose strength is greater in the DGRP group than in the CGRP group, and hollow arrows correspond to the links whose strength is greater in CGRP than in DGRP. This figure shows the results of comparing the effective links between the DGRP and CGRP groups for girls (white circle on the arrow), for boys (black circle on the arrow), and for both genders (white circle with a cross inside).
Рис. 1. Эффективные связи значимо различаются у подростков с признаками девиантного поведения (DGRP) и подростков из контрольной группы (CGRP). Для каждого из шести частотных диапазонов (θ, α1, α2, β1, β2, γ) три RSN – три нейронных сети покоя (CEN, SN, DMN) – показаны в виде овалов. В каждом овале два прямоугольника соответствуют двум узлам сети (двум областям коры). Стрелками показаны направленные взаимодействия (эффективные связи) между разными RSN. Стрелки могут начинаться на контуре овала (RSN как целое оказывает влияние на то, куда указывает конец стрелки) или же на прямоугольнике (влияние оказывает соответствующий узел RSN). Аналогично, стрелки могут заканчиваться на контуре овала (воздействию подвергается вся RSN как целое) или на прямоугольнике (влиянию подвергается узел RSN). Полнотелые стрелки соответствуют связям, которые сильнее в DGRP, чем в CGRP, а пустотелые стрелки – связям, которые, наоборот, сильнее в CGRP, чем в DGRP. Результат сравнения групп DGRP и CGRP показан раздельно для девочек (белый кружок на стрелке), мальчиков (черный кружок на стрелке) и для всех подростков (белый кружок с крестом внутри).
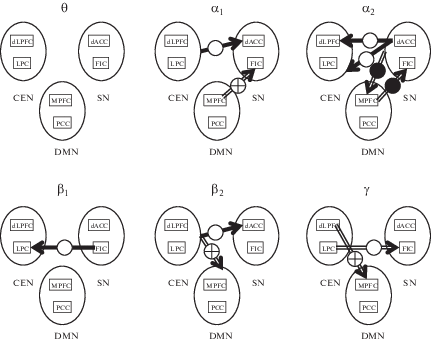
Not that below we report only significant differences, mainly those concerning the difference between DGRP and CGRP groups. If no significant differences in a certain frequency range for a particular analysis and particular gender are present, no mention is given in the text.
CEN-SN connectivity
The analysis of variance of the effective connections between CEN and SN RSNs revealed a number of statistically significant interactions with the GR factor, some of which included the FR factor. Let us consider first all the interactions that are independent of the EEG frequency range. Statistically significant were the following interactions: LOC2 × GR (F(1, 48) = 4.028, p = 0.050, $\eta _{{\text{p}}}^{{\text{2}}}$ = 0.077), LOC2 × GEN × GR (F(1, 48) = = 5.108, p = 0.028, $\eta _{{\text{p}}}^{{\text{2}}}$ = 0.086) and LOC1 × DIR × GEN × GR (F(1, 48) = 4.694, p = 0.035, $\eta _{{\text{p}}}^{{\text{2}}}$ = = 0.075).
The effective connections between CEN and SN includes connections of CEN nodes (dLPFC and LPC) to (1) FIC and (2) to dACC. Paired intergroup comparisons for each of these types of links without taking into account the GEN factor did not reveal any significant differences. Since the three-way interaction LOC2 × GEN × GR turned out to be significant, we tested the effect of the GR factor separately for boys and girls. These statistical tests showed a significantly (F(1, 22) = = 4.704, p = 0.041, $\eta _{{\text{p}}}^{{\text{2}}}$ = 0.176) stronger CEN-to-dACC effective connections in DGRP girls (mean = 0.191 ± 0.064) than CGRP girls (mean = = 0.154 ± 0.032). An analysis of the effective links of each CEN node with dACC in both directions separately in boys and girls did not reveal significant group differences.
There were several high order interactions involving FR factor: FR × LOC1 × GEN × GR (F(5, 44) =2.936, p = 0.023, $\eta _{{\text{p}}}^{{\text{2}}}$ = 0.250), FR × × LOC2 × GEN × GR (F(5, 44) =2.934, p = = 0.023, $\eta _{{\text{p}}}^{{\text{2}}}$ = 0.250) and FR × LOC2 × DIR × GR (F(5, 44) = 4.412, p = 0.002, $\eta _{{\text{p}}}^{{\text{2}}}$ = 0.334). The presence of these interactions indicates the frequency, gender, and topographic specificity of group differences in the effective links between the CEN and SN networks. The GEN factor is involved in all the above interactions except the last one. In this regard, it seemed reasonable to conduct an additional analysis of variance of effective connections for each frequency range separately for girls and boys. This additional analysis revealed the following.
In the alpha-1 range, a significant LOC2 × GR interaction (F(1, 22) = 6.887, p = 0.015, $\eta _{{\text{p}}}^{{\text{2}}}~$ = = 0.238) was found in girls. More detailed analysis of this interaction in girls revealed inter-group differences in effective connections between CEN nodes and dACC node of SN network (F(1, 22) = = 5.856, p = 0.024, $\eta _{{\text{p}}}^{{\text{2}}}$ = 0.210) with greater averaged connection strength in DGRP girls (0.216 ± 0.059) than in their control group counterparts (0.150 ± ± 0.058). Although no significant interactions involving DIR or GR factors were found, still the directed influence of dACC on both CEN nodes was significantly stronger (F(1, 22) = 12.164, p = = 0.002, $~\eta _{{\text{p}}}^{{\text{2}}}$ = 0.356) than the reverse influence.
In boys, no effect of GR factor or any interaction of the latter with other factors was found in alpha-1 frequency range.
Similar intergroup differences were found for the alpha-2 frequency range. In girls, the LOC2 × × GR interaction showed only a tendency toward significance (F(1, 22) = 3.285, p = 0.084, $\eta _{{\text{p}}}^{{\text{2}}}$ = = 0.130), however, the intergroup differences in effective connections between dACC and CEN nodes were significant (F(1, 22) = 4.353, p = = 0.044, $\eta _{{\text{p}}}^{{\text{2}}}$ = 0.159), and stronger averaged effective connection was observed in the DGRP girls (0.233 ± 0.059) than in CGRP girls (0.175 ± 0.058). Again, as it was found for alpha-1, regardless of the group, the dACC-to-CEN connections turned out to be stronger than reverse CEN-to-dACC connections. In apha-2 frequency range, significant differences were found for dACC-to-dLPFC connection (F(1,23) = 33.891, p < 0.0001, $\eta _{{\text{p}}}^{{\text{2}}}$ = 0.596 ) between DGRP and CGRP girls. In boys, no significant intergroup differences were found in effective connectivity between CEN and SN nodes for the alpha-2 frequency band.
In the beta-1 frequency band, we found statistically significant differences between DGRP and CGRP girls in the strength of FIC-to-LPC effective link (F(1, 22) = 4.428, p = 0.047, $\eta _{{\text{p}}}^{{\text{2}}}$ = 0.168), with this link being stronger in DGRP (0.217 ± ± 0.058) than in CGRP (0.154 ± 0.059) girls. Regardless of the group belongership, FIC-to-LPC connection was stronger that the reverse LPC-to-FIC connection (F(1, 22) = 74.242, p < 0.0001, $\eta _{{\text{p}}}^{{\text{2}}}$ = 0.771). No significant intergroup differences were found in boys in this frequency range.
In the beta-2 range, the LOC2 × GR interaction that approached significance was revealed in girls (F(1, 22) = 3.931, p = 0.060, $\eta _{{\text{p}}}^{{\text{2}}}$ = 0.152). This interaction reflected significant intergroup differences that were observed for CEN-to-dACC links (F(1, 22) = 5.632, p = 0.027, $\eta _{{\text{p}}}^{{\text{2}}}$ = 0.204). Stronger effective connections were found in the DGRP girls (0.190 ± 0.029) as compared to the CGRP girls (0.157 ± 0.029). Similar to lower frequency ranges, the dACC-to-CEN links were stronger than the links to the opposite direction (F(1, 22) = 5.875, p = 0.024, $\eta _{{\text{p}}}^{{\text{2}}}$ = 0.210). No significant intergroup differences were found in boys in this frequency range.
In the gamma frequency range, significant interactions of LOC2 × GR (F(1, 22) = 6.812, p = = 0.016, $\eta _{{\text{p}}}^{{\text{2}}}$ = 0.236) and LOC1 × LOC2 × DIR × × GR (F(1, 22) = 8.499, p = 0.008, $\eta _{{\text{p}}}^{{\text{2}}}$ = 0.274) were found in girls. Intergroup comparisons of individual effective connections showed that the impact of the LPC node of the CEN network onto the insular cortex (FIC node of SN network) is stronger in CGRP (0.327 ± 0.082) than in DGRP (0.229 ± 0.108) girls (F(1, 22) = 5.428, p = 0.029, $\eta _{{\text{p}}}^{{\text{2}}}$ = 0.198).
No significant effects were found in boys in the gamma frequency range.
A detailed statistical analysis of effective connectivity between the CEN and SN nodes, which we reported above in this section, showed that significant intergroup differences are observed only in girls. Besides, a common for all frequency ranges is a stronger influence of the dorsal anterior cingulate cortex (dACC) on the CEN nodes in the DGRP girls as compared to their CGRP counterparts. An analysis of variance conducted separately for each frequency range provided additional information on the specifics of effective links between CEN and SN nodes in DGRP girls. In general, the results of these analyses indicate a more pronounced effect of SN nodes on CEN nodes in the DGRP, this applies mainly to the dACC in the alpha-1, alpha-2 and beta-2 frequency ranges. In the beta-1 range, a more pronounced effect of FIC node of SN onto LPC node of CEN was also found. Intergroup differences of the opposite direction (connections being stronger in the control group) were found only in the high-frequency gamma range and limited to the effect of the parietal node of the CEN on the FIC node of SN. In boys, there were no significant differences in the strength of the effective links between the control management network and the key event detection network.
CEN-DMN connectivity
For this pair of RSNs, the following statistically significant interactions involving FR factor were found: FR × LOC1 × DIR × GR (F(5, 44) = = 2.913, p = 0.023, $\eta _{{\text{p}}}^{{\text{2}}}$ = 0.249) and FR × LOC2 × × DIR × GR (F (5, 44) = 2.897, p = 0.024, $\eta _{{\text{p}}}^{{\text{2}}}$ = = 0.248). Taking into account these interactions, further analyses of variance were carried out separately for each frequency range.
Significant intergroup differences were found for the high-frequency beta-2 and gamma frequency bands. In the beta-2 range, the interaction LOC1 × LOC2 × GR approaching statistical significance (F(1, 50) = 3.353, p = 0.073, $\eta _{{\text{p}}}^{{\text{2}}}$ = = 0.065) was found. An analysis of individual effective connections involved in this interaction revealed stronger effective connections between the frontal nodes of CEN and DMN (the lateral and medial areas of the prefrontal cortex) in CGRP (0.115 ± 0.016) than in DGRP (0.104 ± 0.014) adolescents (F(1, 50) = 7.115, p = 0.010, $\eta _{{\text{p}}}^{{\text{2}}}$ = 0.125). In both groups of participants, the impact of MPFC on dLPFC was stronger than the opposite effect (F(1, 50) = 98.420, p < 0.0001, $\eta _{{\text{p}}}^{{\text{2}}}$ = 0.663).
In the gamma frequency range, a three-way LOC1 × LOC2 × GR (F(1, 50) = 4.234, p = = 0.045, $\eta _{{\text{p}}}^{{\text{2}}}$ = 0.078) significant interaction was found. An analysis of individual connections involved in the above interaction revealed higher values of effective connections between frontal nodes (the lateral and medial prefrontal cortex) of the CEN and DMN in the CGRP (0.115 ± 0.016) compared with DGRP (0.105 ± 0.018) adolescents (F(1, 50) = 4.504, p = 0.039, $\eta _{{\text{p}}}^{{\text{2}}}$ = 0.083). At the same time, regardless of the group, the influence of MPFC on dLPFC is stronger than the opposite effect (F(1, 50) = 79.967, p < 0.0001, $\eta _{{\text{p}}}^{{\text{2}}}$ = = 0.615).
Thus, exploring the connections between the nodes of CEN and DMN revealed significant differences between adolescents from the control group and their peers showing signs of deviant behavior. These differences were limited to high-frequency beta-2 and gamma ranges. It also was found that the connection strength between the prefrontal nodes of these networks – the lateral and medial prefrontal cortex (dLPFC and MPFC) – is higher in the control group than in the group adolescents with signs of deviant behavior. It should be noted that for both beta-2 and gamma frequency ranges and regardless of the group belonging, the impact of the medial prefrontal cortex onto the lateral prefrontal cortex is higher than the opposite influence.
SN-DMN connectivity
Similar to what has been found for CEN-SN and SN-DMN pairs, the effective connections between nodes of SN and DMN were different in their strength in different frequency ranges. We found the following two significant gender-nonspecific interactions including the FR and GR factors: FR × LOC2 × GR (F(5, 44) = 3.771, p = = 0.006, $\eta _{{\text{p}}}^{{\text{2}}}$ = 0.300), FR × LOC1 × DIR × GR (F(5, 44) = 2.605, p = 0.038, $\eta _{{\text{p}}}^{{\text{2}}}$ = 0.228). Also, three more interactions additionally involving GEN factor were found: FR × LOC1 × GEN × GR (F(5, 44) = 2.605, p = 0.038, $\eta _{{\text{p}}}^{{\text{2}}}$ = 0.228), FR × × DIR × GEN × GR (F(5, 44) = 4.798, p = = 0.001, $\eta _{{\text{p}}}^{{\text{2}}}$ = 0.353) and FR × LOC1 × LOC2 × × DIR × GEN × GR (F(5, 44) = 2.999, p = = 0.021, $\eta _{{\text{p}}}^{{\text{2}}}$ = 0.254). Since the FR factor has participated in all the above interactions, we performed a separate analysis of variance in each frequency range.
In the alpha-2 frequency range, an interaction LOC1 × LOC2 × DIR × GR (F(1, 48) = 4.004, p = 0.051, $\eta _{{\text{p}}}^{{\text{2}}}$ = 0.077) that approached a statistical significance was found. Paired group comparisons of individual effective connections of both directions revealed a significantly stronger effect of the medial prefrontal cortex, MPFC, on the insular cortex, FIC, in the CGRP (0.164 ± 0.055) than DGRP (0.132 ± 0.051) adolescents (F(1, 50) = = 4.364, p = 0.042, $\eta _{{\text{p}}}^{{\text{2}}}$ = 0.080). In addition, a significant LOC2 × GEN × GR (F(1, 48) = 5.190, p = 0.027, $\eta _{{\text{p}}}^{{\text{2}}}$ = 0.098) interaction was found, which was caused by stronger effective links between the SN nodes and MPFC (F(1, 26) = 5.147, p = 0.032, $\eta _{{\text{p}}}^{{\text{2}}}$ = 0.165) in boys of the control group (0.171 ± 0.035) than in the DGRP boys (0.141 ± ± 0.036). Intergroup comparisons of effective connections in individual pairs of nodes revealed a stronger effect of MPFC on FIC (F(1, 26) = = 4.500, p = 0.042, $\eta _{{\text{p}}}^{{\text{2}}}$ = 0.149) in CGRP boys (0.170 ± 0.059) than in DGRP boys (0.124 ± 0.052). No significant intergroup differences, however, were found in girls.
Thus, significant intergroup differences in the links between nodes of the salience network and nodes of the default mode network were identified only for the alpha-2 frequency range: namely, the influence of MPFC node of the default mode network onto the FIC node of SN was stronger in CGRP than in DGRP adolescents, with this differences being more pronounced in boys than in girls.
DISCUSSION
The results of the performed statistical analysis indicate that at rest, the pattern of effective connections between the cortical regions belonging to CEN, SN and DMN differs in DGRP and CGRP adolescents. Moreover, the differences between the groups turned out to be frequency-specific and unequal in girls and boys.
For a neurophysiological interpretation of the results, it is necessary to take into account that the adolescence is a period of rapid structural changes in the brain [Arain et al., 2013]. The structural changes occurring in the major focal points of communication or interaction in the brain, network hubs, seem to play a particularly noticeable role [Cole et al., 2013]. The genetically patterned process of the hub formation and consolidation is protracted and extends into late adolescence [Oldham, Fornito, 2019]. This process is characterized by a high degree of individual variability and carries the risks of anomalies [Whitaker et al., 2016]. Application of the graph theory measures to human connectome data [Mišic et al., 2016; Sporns, 2018] showed that FIC and dACC nodes can be viewed as hubs [Sato et al., 2016; Cai et al., 2016].
As noted in the introduction, several models have been proposed that explain the characteristics of adolescence [Casey, 2015; Shulman et al., 2016]. Essentially, these models are based on the same general idea of a developmental imbalance between the activity of brain structures that underlie emotional and motivational functions, on the one hand, and control functions, on the other. In particular, this imbalance can be directly related to difference in the maturation course of various brain structures: subcortical regions closely associated with emotional-motivational processes mature earlier than the prefrontal cortex related to executive functions. This mismatch may result in the elevated rate of risk-taking and sensation-seeking behaviors observed during adolescence.
The presence of such mismatch is experimentally confirmed by tracing individual development paths. For example, a follow-up study [Mills et al., 2014] of individual trajectories (late childhood, adolescence and early adulthood) of the brain structural maturation by measuring gray matter volume showed relatively earlier maturation of the amygdala and nucleus accumbens compared to prefrontal cortex. Interestingly, that an attempt to link these individual development trajectories with a retrospective self-assessment of risky behavior and sensation-seeking behaviors during adolescence was not successful. Although, this failure could be attributed to the inaccuracy of the retrospective assessment of one’s own behavior, another reason for it seems plausible: when assessing the degree of maturity, it is necessary to take into account not only the state of gray matter, but also the degree of maturity of the pathways.
The most direct confirmation of the importance of connections between brain structures (not just the degree of maturity of the structures themselves) is the tractography data. Adolescents prone to risky activities have been shown to have more mature white matter characteristics (more adult-like) than their more cautious peers [Berns et al., 2009]. This seemingly paradoxical result shows that “more mature” does not always mean “better” and that, in full accordance with theoretical concepts, the lack of bias in the development of individual brain structures is more important for the organization of behavior than the absolute level of their maturity.
The data reported in the present paper directly supports the view according to which inter-regional connections are of utmost importance for brain functioning and therefore for observed behaviors. Note that adolescents belonging to DGRP are not those suffering from any gross pathology but those labeled by their parents and/or teachers as showing socially unacceptable behavior. Yet, even this clinically mild departure turned out to be enough to reveal the connectivity pattern different from that of the control group.
What roles do the DMN, SN CEN nodes play, and why are their state and the connections between them so important for the organization of behavior?
The structures that make up DMN are involved in processes related to autobiographical memory and introspective thought activity [Catani et al., 2013] and closely related to the network nodes of SN and CEN [Chen et al., 2013]. Importantly, the interaction between SN, CEN, and DMN is critical to ensure rapid switching between different tasks [Sridharan et al., 2008]. The SN structures, which serve to detect salient events, have to activate CEN, which serves to organize arbitrary attention, and at the same time have to suppress DMN activity, reflecting self-referenced processes. It is the dorsal anterior insula that is in a perfect position to integrate external sensory information with internal emotional and bodily state signals to coordinate brain network dynamics and to initiate switches between the DMN and CEN [Uddin et al., 2017]. Also, anterior right insula plays a central role in the SN response to errors [Ham et al., 2013]. This structure serves as the input through which information is received about errors that arise when solving problems requiring attention. Besides, effective links between FIC and dACC are of importance for ongoing adjustments to cognitive control of behavioral responses.
The cingulate cortex and amygdala are involved in a number of sensory and cognitive processes, as well as emotionally motivational processes. The optimal state of the connections between the amygdala and the cingulate cortex is an essential precondition for the effective regulation of emotions in the limbic system [Bernhardt, Singer, 2012]. Therefore, we can expect that changes in the character/strength of such connections can affect the cortical-cortical connections between the cingulate and prefrontal cortices (i.e. the links between DMN and CEN) we observe experimentally. An indirect support for that view came from the study that showed that individuals who are more successful in the voluntary regulation of their emotional states (measured by cognitive reappraisal ability) are also characterized by less anxiety and more positive daily emotions at the behavioral level, and by greater activation in the medial and lateral prefrontal areas and at rest with lesser strength of functional connections between the right amygdala, on the one hand, and the medial prefrontal cortex and posterior cingulate cortex, on the other [Uchida et al., 2015].
The potentially significant role of the non-optimal state (such as immaturity or degradation) of the six studied cortical structures and the connections between them in the occurrence of deviant behavior is directly indicated by adolescent behaviors and indirectly by clinical data.
The existence of a correlation between the propensity to risk-taking and the strength of the functional relationships of MPFC with other brain structures has been experimentally confirmed [Qu et al., 2015]. In particular, it was shown that the degree of decrease in the strength of the functional connection between the medial prefrontal cortex (MPFC) and the ventral striatum over a year and a half is statistically related to the decrease in the risky behavior reported by the subjects.
The functional connections of the right and left amygdala with the dorsomedial and ventromedial prefrontal cortex (at least in women) reveal a selective statistical relationship with such a temperament characteristic as “harm avoidance” [Baeken et al., 2014]. In particular, higher values of the harm avoidance scale corresponded to stronger functional connections of the right amygdala with the dorsomedial prefrontal cortex (dmPFC), which is involved in the regulation of negative emotions. In addition, the cited work directly demonstrated the presence of a statistical relationship between the activity of the cingulate cortex and the “harm avoidance” scale.
The relationship between low-frequency fluctuations of the BOLD signal at rest and a tendency to impulsive behavior (negative urgency (NU) and positive urgency (PU)), which is one of the symptoms of inadequately risky behavior (for example, addiction and causing bodily harm) was studied in adult subjects [Zhao et al., 2017]. It has been shown that even in healthy volunteers, the NU and PU values are statistically associated with fluctuations of the BOLD signal in the lateral frontal orbital cortex, dorsolateral prefrontal cortex, dorsomedial and ventral frontal cortex, as well as the anterior and posterior cingulate cortex. In addition, the NU and PU indices found a significant correlation with the strength of the functional connection of the posterior cingulate cortex with the thalamus. Experimental data have also been obtained that indicate the presence of a correlation between the drug addiction and the decreased strength of the functional connection between the amygdala and the insula; decreased strength of the functional connections are also found between the anterior cingulate cortex and the ventromedial prefrontal cortex, on the one hand, and the nodes of the CEN network, on the other [Wilcox et al., 2016].
There are a large body of clinical data showing an altered interactions of the brain structures directly included in or tightly related to DMN, CEN, SN. In particular, the anterior insula is susceptible to pathological processes occurring in frontotemporal dementia (FTD), most notably in behavioral variant FTD (bvFTD), in which gray matter loss in frontal and anterior insular regions has been observed. Poor judgment, impulsivity, and lack of empathy are clinical symptoms of bvFTD which are consistent with the known functions of the anterior insula [Shura et al., 2014]. Numerous studies showed that adolescents with clinical depression were characterized by an altered connectivity of the CEN, DMN, and SN nodes. For example, it has been shown that in adolescents with clinical depression, the nature of the functional relationships between FIC and LPC at rest differs from that in adolescents in the control group [Pannekoek et al., 2014]. Adolescents with clinical depression showed an increase in the strength of the functional connections of the left amygdala with the right parietal cortex, while the strength of such connections of the right amygdala with the left frontal areas of the cortex, including ACC, decreased. A decrease in the strength of functional connections between dACC (bilateral) with the right middle frontal gyrus, frontal pole and lower frontal gyrus in clinically depressed adolescents also was found. It was found that the local connectivity of the dorsal medial prefrontal cortex was reduced during self-reflection in depressed adolescents [Bradley et al., 2016]. In adolescents with depression, the negative inhibitory impact of MPFC on PCC in the process of self-assessment increased significantly more than in adolescents in the control group [Davey et al., 2017]. It was also shown that the strength of modulation of MPFC-PCC effective connectivity measured using dynamic causal modeling (DCM) was negatively correlated with a Montgomery-Asberg Depression Rating Scale factor that reflected difficulties with concentration and inner tension. This result, which is pointing to the “hyperregulatory” impact of MPFC onto the posterior cingulate cortex in depressed adolescents, is an experimental confirmation of the significant role of connections in ensuring the functional properties of distributed brain systems that ultimately determine behavior in including clinical conditions.
The results presented in this study are generally consistent with the above literature data. We showed that two groups of adolescents, DGRP and CGRP, show different patterns of effective links between the DMN, SN, and CEN nodes. However, given the current level of understanding of the mechanisms of interaction among the DMN, SN, and CEN structures, it is difficult to ascertain why changes in certain directed influences of some structures on others lead to observed behavioral and clinical consequences.
Difficulties in interpreting the data that we mention above become clear when trying to account for the gender-related differences in the pattern of effective relationships. In principle, the effect of adolescent sex on the strength of functional and effective connections at rest and in the process of activity is quite expected and experimentally confirmed [Satterthwaite et al., 2015; de Lacy et al., 2019]. However, if we focus on the connections within and between DMN, SN and CEN, then the picture gets far from unambiguous. For example, when studying age-related changes in the strength of functional and effective in these three RSNs, the influence of gender on the strength of effective bonds was found in the high-frequency ranges beta-2 (20–27 Hz) and gamma (30–40 Hz) in a group of teenagers aged 11–13 years [Machinskaya et al., 2019]. This influence manifests itself in stronger links within DMN and CEN in girls compared with boys. On the other hand, the study of a number of RSNs in a wide age range from 7 to 18 years, including in DMN, CEN and SN, showed that in all three RSNs there is a change in the strength of the functional connection (in the low frequency region of the BOLD signal) and in particular, increase in strength was observed for fronto-parietal links in CEN, and for links between the prefrontal and anterior cingulate cortex in SN [Solé-Padullés et al., 2016]. However, the influence of gender on functional relationships was not found in this work.
Nevertheless, we believe that there are good reasons to consider the development paths of the links within the three discussed RSNs different for the two genders. One of these reasons is sexual dimorphism in the maturation and functional connections of the amygdala – a structure that takes part in the processing of emotions, decision-making and is closely related to the DMN and SN networks considered in our study. Amygdala maturation proceeds differently in girls and boys: while adolescent girls show a relatively small change in the amount of amigdala gray matter, in boys, the amygdala volume continues to increase significantly up to 18 years of age [Brenhouse, Andersen, 2011]. Besides, the functional connections of the amygdala differ in men and women and depend on the level of cortisol [Kogler et al., 2016].
The studies of age-related changes in functional and effective connectivity give us insights into the general direction of these changes. In particular, the age-related changes in the DMN and CEN have been studied in a longitudinal sample of 45 adolescents aged 10 and 13 [Sherman et al., 2014]. According to this work, two tendencies are observed during the age period from 10 to 13 years: the tendency to increase the strength of within-RSN connections as well as the tendency to decrease the connection strength between-RSN (increasing segregation between networks). From this point of view, the seemingly stronger connections between the CEN and SN nodes in the alpha and beta frequency ranges in girls of their DGRP compared to their peers from CGRP found in our study could be attributed to the general delay in maturation in girls with deviant behavior. However, this explanation is in contradiction with the weaker links between CEN and DMN observed in the DGRP group (in both girls and boys), namely, the reduced strength of reciprocal bonds between dLPFC and MPFC in the high-frequency ranges (beta2 and gamma).
In addition to the general difficulties that arise in interpreting our results and are associated with limited knowledge of the detailed structure and functioning of brain systems, there are also difficulties associated with the specifics of the present study, which is subject to a number of limitations.
First, we did not use the correction for multiple statistical tests. This was done on purpose, because our study was exploratory in nature, and we considered it more harmful to reject a possible effect (type II error) than finding a false effect (type I error). The downside of this strategy however is the presence of a number of spurious differences.
Second source of limitations is the use of a fundamentally linear vector autoregressive modeling. VAR modeling allows to estimate frequency-specific directed influence between cortical sources of EEG via directed coherence function but, unlike DCM, it does not allow us to distinguish between excitatory and inhibitory connections.
Finally, since we did not use the individual MRI scans and electrode placements while computing gain matrix, the resultant distribution of the source activity across voxels might have been shifted away from its actual position. In part this effect was offset by using the first principal component representing a signal common to several voxels confined within a 10 mm sphere around the ROI center.
CONCLUSIONS
An analysis of the differences between DGRP and CGRP adolescents in the strength of the effective interaction between the nodes of the central executive network (CEN), the salience network (SN) and the default mode network (DMN) in different EEG frequency ranges showed the following.
1. A significant gender effect on the pattern of the cortical-cortical interaction between the rest networks is observed. The differences between DGRP and CGRP adolescents in the connections between CEN and SN were found mainly in girls, the links between CEN and DMN are not affected by gender, while the differences in SN-to-DMN connections were observed in boys.
2. Intergroup differences in the strength of effective connections between CEN, SN, and DMN are frequency-specific: for CEN and SN links, they were mainly observed in the alpha and beta frequency bands, for links between CEN and DMN they were found in beta-2 and gamma frequency bands, and for links between SN and DMN they were detected in alpha-2 frequency range.
3. When compared with girls from the control group, a characteristic feature of effective connections in girls with signs of deviant behavior is the stronger impact of the dorsal anterior cingulate cortex onto the CEN nodes of the central executive network, and at the same time the weaker influence of the parietal CEN node onto the insular cortex.
4. In adolescents with difficulties in regulating behavior, effective connections between the prefrontal nodes of CEN and DMN, i.e. those between the right dorsolateral prefrontal and medial prefrontal cortex, are reduced compared to adolescents in the control group.
5. In adolescents with difficulties in regulating behavior, the influence of the prefrontal node of the default mode network, the medial prefrontal cortex, onto the cortex of the frontal part of the insular cortex is weaker than in the control group.
Список литературы
Arain M., Haque M., Johal L., Mathur P., Nel W., Rais A., Sandhu R., Sharma S. Maturation of the adolescent brain. Neuropsychiatr Dis Treat. 2013. 9: 449–461.
Baccala L.A., Sameshima K., Ballester G., Do Valle A.C., Timo-Iaria C. Studying the interaction between brain structures via directed coherence and Granger causality. Appl. Signal Processing. 1998. 5: 40–48.
Baeken C., Marinazzo D., Van Schuerbeek P., Wu G.R., De Mey J., Luypaert R., De Raedt R. Left and right amygdala – mediofrontal cortical functional connectivity is differentially modulated by harm avoidance. PLoS One. 2014. 9 (4): e95740.
Bernhardt B.C., Singer T. The neural basis of empathy. Annu Rev Neurosci. 2012. 35: 1–23.
Berns G.S., Moore S., Capra C.M. Adolescent engagement in dangerous behaviors is associated with increased white matter maturity of frontal cortex. PLoS One. 2009. 4 (8): e6773.
Botdorf M., Rosenbaum G.M., Patrianakos J., Steinberg L., Chein J.M. Adolescent risk-taking is predicted by individual differences in cognitive control over emotional, but not non-emotional, response conflict. Cogn Emot. 2017. 31 (5): 972–979.
Bradley K.A., Colcombe S., Henderson S.E., Alonso C.M., Milham M.P., Gabbay V. Neural correlates of self-perceptions in adolescents with major depressive disorder. Dev Cogn Neurosci. 2016. 19: 87–97.
Brenhouse H.C., Andersen S.L. Developmental trajectories during adolescence in males and females: a cross-species understanding of underlying brain changes. Neurosci Biobehav Rev. 2011. 35 (8): 1687–1703.
Bressler S.L., Menon V. Large-scale brain networks in cognition: emerging methods and principles. Trends Cogn Sci. 2010. 14 (6): 277–290.
Cai W., Chen T., Ryali S., Kochalka J., Li C.S., Menon V. Causal Interactions Within a Frontal-Cingulate-Parietal Network During Cognitive Control: Convergent Evidence from a Multisite-Multitask Investigation. Cereb Cortex. 2016. 26 (5): 2140–2153.
Caouette J.D., Feldstein Ewing S.W. Four Mechanistic Models of Peer Influence on Adolescent Cannabis Use. Curr Addict Rep. 2017. 4 (2): 90–99.
Casey B.J. Beyond simple models of self-control to circuit-based accounts of adolescent behavior. Annu Rev Psychol. 2015. 66: 295–319.
Casey B.J., Getz S., Galvan A. The adolescent brain. Dev Rev. 2008. 28 1): 62–77.
Catani M., Dell’acqua F., Thiebaut de Schotten M. A revised limbic system model for memory, emotion and behaviour. Neurosci Biobehav Rev. 2013. 37 (8): 1724–1737.
Che D., Hu J., Zhen S., Yu C., Li B., Chang X., Zhang W. Dimensions of Emotional Intelligence and Online Gaming Addiction in Adolescence: The Indirect Effects of Two Facets of Perceived Stress. Front Psychol. 2017. 8: 1206.
Chen A.C., Oathes D.J., Chang C., Bradley T., Zhou Z.W., Williams L.M., Glover G.H., Deisseroth K., Etkin A. Causal interactions between fronto-parietal central executive and default-mode networks in humans. Proc Natl Acad Sci U S A. 2013. 110 (49): 19944–19949.
Cole M.W., Reynolds J.R., Power J.D., Repovs G., Anticevic A., Braver T.S. Multi-task connectivity reveals flexible hubs for adaptive task control. Nat Neurosci. 2013. 16 (9): 1348–1355.
Cui J., Xu L., Bressler S.L., Ding M., Liang H. BSMART: A Matlab/C toolbox for analysis of multichannel neural time series. Neural Networks. 2008. 21 (8): 1094–1104.
Darvas F., Pantazis D., Kucukaltun-Yildirim E., Leahy R.M. Mapping human brain function with MEG and EEG: methods and validation. Neuroimage. 2004. 23 Suppl 1: S289–299.
Davey C.G., Breakspear M., Pujol J., Harrison B.J. A brain model of disturbed self-appraisal in depression. Am J Psychiatry. 2017. 174 (9): 895–903.
de Lacy N., McCauley E., Kutz J.N., Calhoun V.D. Multilevel mapping of sexual dimorphism in intrinsic functional brain networks. Front Neurosci. 2019. 13: 332.
Diamond A. Executive functions. Annu Rev Psychol. 2013. 64: 135–168.
Ernst M., Pine D.S., Hardin M. Triadic model of the neurobiology of motivated behavior in adolescence. Psychol Med. 2006. 36 (3): 299–312.
Esménio S., Soares J.M., Oliveira-Silva P., Zeidman P., Razi A., Gonçalves Ó.F., Friston K., Coutinho J. Using resting-state DMN effective connectivity to characterize the neurofunctional architecture of empathy. Sci Rep. 2019. 9: 2603.
Fox M.D., Raichle M.E. Spontaneous fluctuations in brain activity observed with functional magnetic resonance imaging. Nat Rev Neurosci. 2007. 8 (9): 700–711.
Franzmeier N., Göttler J., Grimmer T., Drzezga A., Áraque-Caballero M.A., Simon-Vermot L., Taylor A.N.W., Bürger K., Catak C., Janowitz D., Müller C., Duering M., Sorg C., Ewers M. Resting-state connectivity of the left frontal cortex to the default mode and dorsal attention network supports reserve in mild cognitive impairment. Front Aging Neurosci. 2017. 9: 264.
Ham T., Leff A., de Boissezon X., Joffe A., Sharp D.J. Cognitive control and the salience network: an investigation of error processing and effective connectivity. J Neurosci. 2013. 33 (16): 7091–7098.
Ho T.C., Connolly C.G., Henje Blom E., LeWinn K.Z., Strigo I.A., Paulus M.P., Frank G., Max J.E., Wu J., Chan M., Tapert S.F., Simmons A.N., Yang T.T. Emotion-Dependent Functional Connectivity of the Default Mode Network in Adolescent Depression. Biol Psychiatry. 2015. 78 (9): 635–646.
Kogler L., Müller V.I., Seidel E.M., Boubela R., Kalcher K., Moser E., Habel U., Gur R.C., Eickhoff S.B., Derntl B. Sex differences in the functional connectivity of the amygdalae in association with cortisol. Neuroimage. 2016. 134: 410–423.
Kurgansky A.V. Study of cortico-cortical functional connectivity with vector autoregressive model of multichannel EEG. Zh Vyssh Nerv Deiat Im I P Pavlova. 2010. 60(6): 740–759. < Курганский А.В. Некоторые вопросы исследования кортико-кортикальных функциональных связей с помощью векторной авторегрессионной модели многоканальной ЭЭГ. Журн. высш. нервн. деят. им. И.П. Павлова. 2010. 60 (6): 740–759.
Litvak V., Mattout J., Kiebel S., Phillips C., Henson R., Kilner J., Barnes G., Oostenveld R., Daunizeau J., Flandin G., Penny W., Friston K. EEG and MEG data analysis in SPM8. Comput Intell Neurosci. 2011. 2011: 852961.
Machinskaya R.I., Kurgansky A.V., Lomakin D.I. Age-related trends in functional organization of cortical parts of regulatory brain systems in adolescents: an analysis of resting-state networks in the EEG source space. Human Physiology. 2019. 45 (5): 461–473. Russian Text © The Author(s), 2019, published in Fiziologiya Cheloveka, 2019, Vol. 45, No. 5, pp. 5–19.
McCormick E.M., Perino M.T., Telzer E.H. Not just social sensitivity: Adolescent neural suppression of social feedback during risk taking. Dev Cogn Neurosci. 2018. 30: 134–141.
McClure S.M., Laibson D.I., Loewenstein G., Cohen J.D. Separate neural systems value immediate and delayed monetary rewards. Science. 2004. 306 (5695): 503–507.
Menon V. Salience Network. In: Arthur W. Toga, editor. Brain Mapping: An Encyclopedic Reference. 2015. vol. 2. pp. 597–611. Academic Press: Elsevier.
Mills K.L., Goddings A.L., Clasen L.S., Giedd J.N., Blakemore S.J. The developmental mismatch in structural brain maturation during adolescence. Dev Neurosci. 2014. 36 (3–4): 147–160.
Mišić B., Betzel R.F., de Reus M.A., van den Heuvel M.P., Berman M.G., McIntosh A.R., Sporns O. Network-Level Structure-Function Relationships in Human Neocortex. Cereb Cortex. 2016. 26 (7): 3285–3296.
Oldham S., Fornito A. The development of brain network hubs. Dev Cogn Neurosci. 2019. 36: 100607.
Pannekoek J.N., van der Werff S.J., Meens P.H., van den Bulk B.G., Jolles D.D., Veer I.M., van Lang N.D., Rombouts S.A., van der Wee N.J., Vermeiren R.R. Aberrant resting-state functional connectivity in limbic and salience networks in treatment–naïve clinically depressed adolescents. J Child Psychol Psychiatry. 2014. 55 (12): 1317–1327.
Pascual-Marqui R.D., Lehmann D., Koukkou M., Kochi K., Anderer P., Saletu B., Tanaka H., Hirata K., John E.R., Prichep L., Biscay-Lirio R., Kinoshita T. Assessing interactions in the brain with exact low-resolution electromagnetic tomography. Philos Trans A Math Phys Eng Sci. 2011. 369 (1952): 3768–3784.
Pessoa L. Understanding emotion with brain networks. Curr Opin Behav Sci. 2018. 19: 19–25.
Qu Y., Galvan A., Fuligni A.J., Lieberman M.D., Telzer E.H. Longitudinal Changes in Prefrontal Cortex Activation Underlie Declines in Adolescent Risk Taking. J Neurosci. 2015. 35 (32): 11308–11314.
Sato J.R., Biazoli C.E. Jr., Salum G.A., Gadelha A., Crossley N., Vieira G., Zugman A., Picon F.A., Pan P.M., Hoexter M.Q., Anés M., Moura L.M., Del’Aquilla M.A., Junior E.A., Mcguire P., Rohde L.A., Miguel E.C., Bressan R.A., Jackowski A.P. Connectome hubs at resting state in children and adolescents: Reproducibility and psychopathological correlation. Dev Cogn Neurosci. 2016. 20: 2–11.
Satterthwaite T.D., Wolf D.H., Roalf D.R., Ruparel K., Erus G., Vandekar S., Gennatas E.D., Elliott M.A., Smith A., Hakonarson H., Verma R., Davatzikos C., Gur R.E., Gur R.C. Linked Sex Differences in Cognition and Functional Connectivity in Youth. Cereb Cortex. 2015. 25 (9): 2383–2394.
Sherman L.E., Rudie J.D., Pfeifer J.H., Masten C.L., McNealy K., Dapretto M. Development of the default mode and central executive networks across early adolescence: a longitudinal study. Dev Cogn Neurosci. 2014. 10: 148–159.
Shulman E.P., Smith A.R., Silva K., Icenogle G., Duell N., Chein J., Steinberg L. The dual systems model: Review, reappraisal, and reaffirmation. Dev Cogn Neurosci. 2016. 17: 103–117.
Shura R.D., Hurley R.A., Taber K.H. Insular cortex: structural and functional neuroanatomy. J Neuropsychiatry Clin Neurosci. 2014. 26 (4): 276–282.
Sockeel S., Schwartz D., Pélégrini-Issac M., Benali H. Large-Scale Functional Networks Identified from Resting-State EEG Using Spatial ICA. PLoS One. 2016. 11 (1): e0146845.
Solé-Padullés C., Castro-Fornieles J., de la Serna E., Calvo R., Baeza I., Moya J., Lázaro L., Rosa M., Bargalló N., Sugranyes G. Intrinsic connectivity networks from childhood to late adolescence: Effects of age and sex. Dev Cogn Neurosci. 2016. 17: 35–44.
Sporns O. Graph theory methods: applications in brain networks. Dialogues Clin Neurosci. 2018. 20 (2): 111–121.
Sridharan D., Levitin D.J., Menon V. A critical role for the right fronto-insular cortex in switching between central-executive and default-mode networks. Proc Natl Acad Sci U S A. 2008. 105(34): 12569–12574.
Steinberg L., Chein J.M. Multiple accounts of adolescent impulsivity. Proc Natl Acad Sci U S A. 2015. 112 (29): 8807–8808.
Uchida M., Biederman J., Gabrieli J.D., Micco J., de Los Angeles C., Brown A., Kenworthy T., Kagan E., Whitfield-Gabrieli S. Emotion regulation ability varies in relation to intrinsic functional brain architecture. Soc Cogn Affect Neurosci. 2015. 10 (12): 1738–1748.
Uddin L.Q., Nomi J.S., Hébert-Seropian B., Ghaziri J., Boucher O. Structure and Function of the Human Insula. J Clin Neurophysiol. 2017. 34 (4): 300–306.
van den Heuvel M.P., Mandl R.C., Kahn R.S., Hulshoff Pol H.E. Functionally linked resting-state networks reflect the underlying structural connectivity architecture of the human brain. Hum Brain Mapp. 2009. 30 (10): 3127–3141.
Whitaker K.J., Vértes P.E., Romero-Garcia R., Váša F., Moutoussis M., Prabhu G., Weiskopf N., Callaghan M.F., Wagstyl K., Rittman T., Tait R., Ooi C., Suckling J., Inkster B., Fonagy P., Dolan R.J., Jones P.B., Goodyer I.M.; NSPN Consortium, Bullmore E.T. Adolescence is associated with genomically patterned consolidation of the hubs of the human brain connectome. Proc Natl Acad Sci U S A. 2016. 113 (32): 9105–9110.
Wilcox C.E., Pommy J.M., Adinoff B. Neural Circuitry of Impaired Emotion Regulation in Substance Use Disorders. Am J Psychiatry. 2016. 173 (4): 344–361.
Yuan H., Ding L., Zhu M., Zotev V., Phillips R., Bodurka J. Reconstructing Large-Scale Brain Resting-State Networks from High-Resolution EEG: Spatial and Temporal Comparisons with fMRI. Brain Connect. 2016. 6 (2): 122–135.
Zhao J., Tomasi D., Wiers C.E., Shokri-Kojori E., Demiral S.B., Zhang Y., Volkow N.D., Wang G.J. Correlation between traits of emotion-based impulsivity and intrinsic default-mode network activity. Neural Plast. 2017. 2017: 9297621.
Дополнительные материалы отсутствуют.
Инструменты
Журнал высшей нервной деятельности им. И.П. Павлова